Client story
The Defence and Science Technology Laboratory (Dstl) set a seriously tough challenge… to develop next generation systems to respond autonomously to a cybersecurity attack on military systems. The multidisciplinary CC team responded by applying a truly leading-edge AI solution and demonstrating a proof of concept in a challenging application.
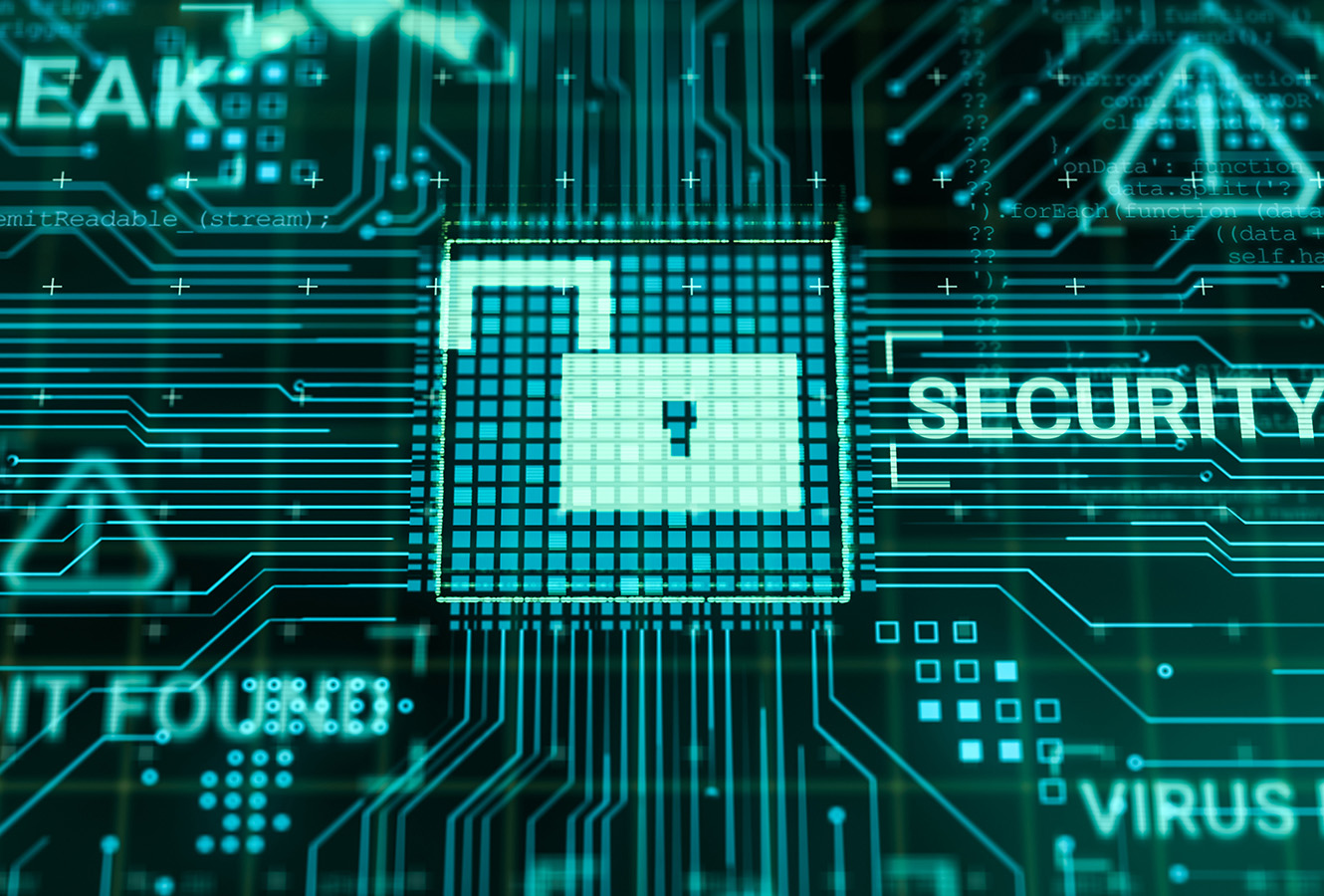
An autonomous cyberattack challenge
Dstl is a department within the UK Ministry of Defence (MOD). As part of the Autonomous Resilient Cyber Defence (ARCD) project, it challenged industry to meet its ambition to reduce impact on the military by establishing an autonomous cyberattack response. The imperative was to exceed technologies which use artificial intelligence to spot anomalies that might indicate attack. Instead, the solution should actually decide the best response.
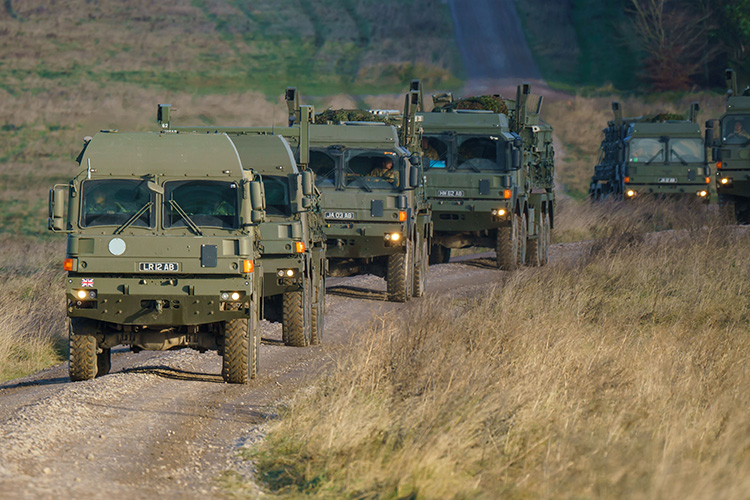
Multi-agent reinforcement learning
The three-year ARCD project aims to research and develop self-defending, self-recovering concepts for military platforms and technologies that can be tested and evaluated against attacks in a representative military environment. For our initiative, we developed a proof of concept using collaborative multi-agent reinforcement learning (MARL) agents – an approach that is currently the subject of much academic research.
“Dstl is pleased to be working with Cambridge Consultants to help us meet the difficult challenge of autonomous cyber defence, delivering cutting-edge response and recovery concepts over the next three years, with the potential to help transform cyber resilience for MOD.”
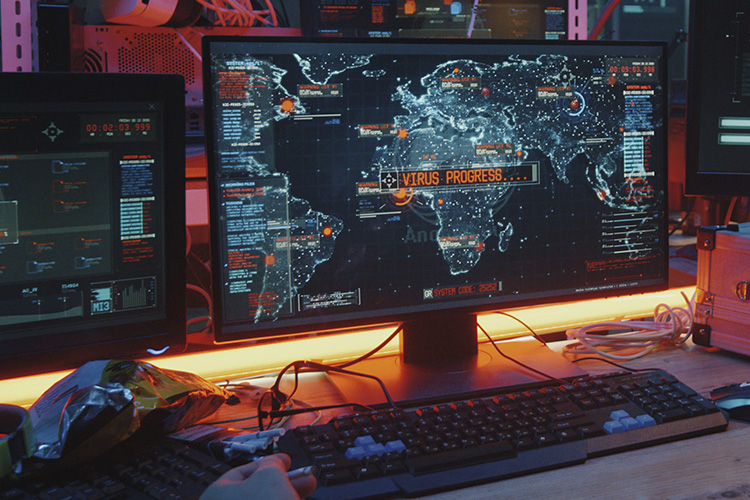
Enabling an autonomous response
There are lots of ways to respond to a cyberattack. Choosing the right one depends on both the attack and the situation – which is not a combination well suited to most AI approaches. But with collaborative MARL, the complex problem of deciding the best response can be broken down into individual decisions. Each one of the multiple learning agents recommends whether their action is appropriate to a particular situation. The agents then collaborate to decide on the best course – which enables an autonomous response to the attack.
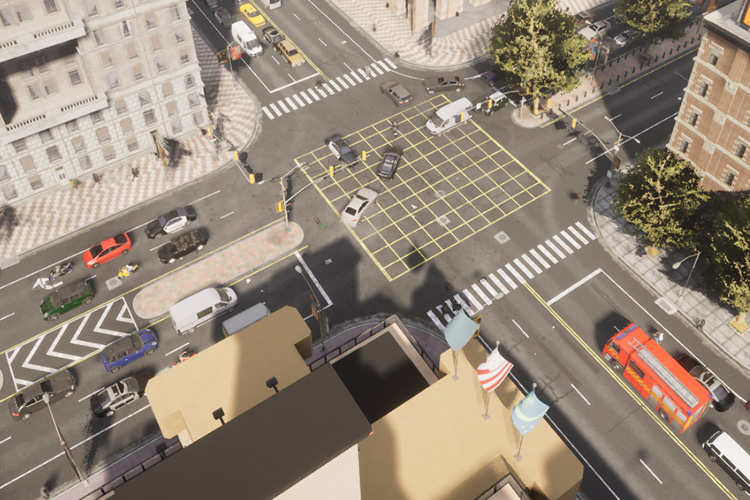
Context defines the correct action
The application we chose to explore was the defence of vehicles, where context is a key part of the problem. This simplified scenario illustrates the challenge: When under a cyberattack, do you stop your vehicle, or is it safer to carry on? The answer depends on the situation. You don’t always slam your brakes on in the middle of a busy road junction because there might be a safer option. This is equally true for a military situation where the context will, in part, define the correct action.
“Developing a system that has the potential to make decisions to respond autonomously to a cyberattack is a complex problem requiring the application of the very latest technologies.”
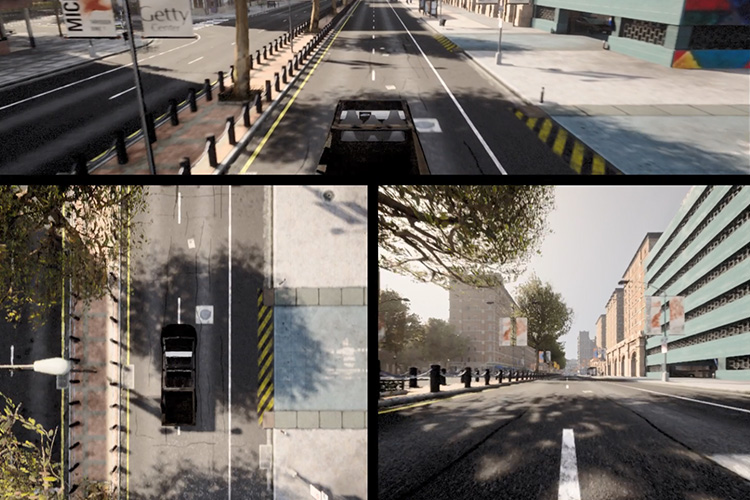
Learning in a safe, simulated environment
Our proof of concept used a simulated environment to train our many AI agents. It meant they could learn from their mistakes in a safe environment. Using simulation also enables us to test as yet unseen cybersecurity attacks and others where real world data is in short supply. We were also able to run simulations faster than real time – so speeding up the training process for the AI.
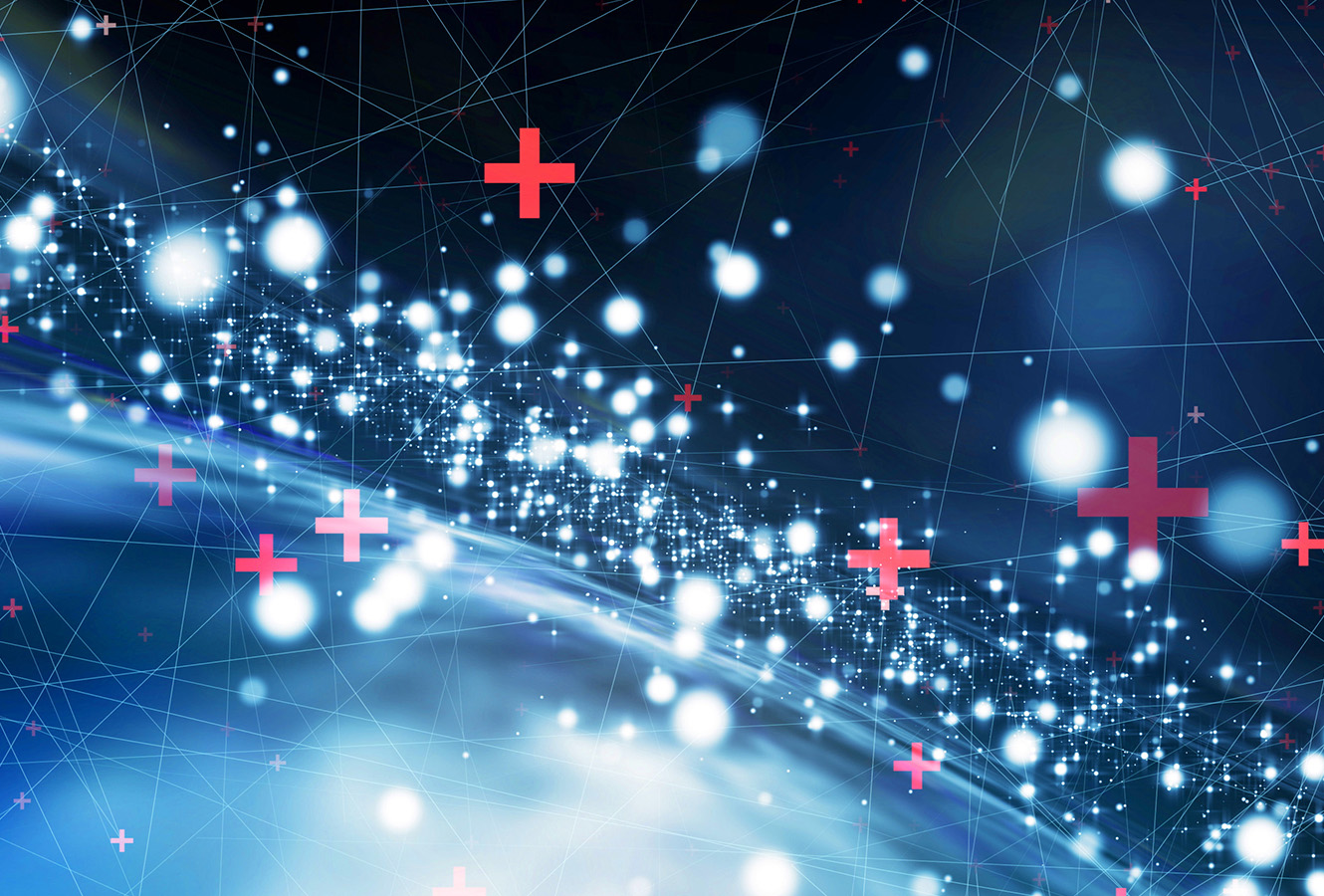
Protecting against wider threats
So where do we go from here? We continue to work with Dstl on research and development of the system. We intend to explore how to better train multiple agents working in parallel, and how to maximise the robustness of the system and so protect against a wider set of threats. Our aim for three years hence is to deliver a demonstration in representative context.