We are currently working on the first systems capable of actually understanding people. I know, that’s a humdinger of a sentence to open with isn’t it. The extraordinary potential of what we might term empathic technology promises rich human/machine relationships that are almost unimaginable. Yet here we are at Cambridge Consultants embarking on practical, encouraging experiments that are taking us further down the road towards emotionally and socially intelligent systems, products and services.
The journey is being navigated by our Human-Machine Understanding (HMU) group, a dedicated in-house team of engineers, scientists and digital designers working to integrate the latest thinking in psychology, artificial intelligence and sensing into tangible outputs. You might have already caught up with my article in which I wrestle with complex challenges such as context understanding, personalised machine learning models and real time insight into the human state.
In this piece, I plan to put a little more flesh on the bone by sharing some insights into our research. But before I get into that, it’s probably worth dealing with a very reasonable question – what’s the point of it all? Well, look at it this way. AI has already advanced incredibly and in countless ways, from solving well-nigh impossible scientific riddles to enabling drug discovery in record time. But humans are emotional to the core and if technology doesn’t ‘get’ our multidimensional nature, there’s a limit to how much better it can get at supporting us. If, or rather when, HMU unshackles technology from this restraint, everything changes.
Human-Machine Understanding programme
So, let’s start to burrow into the detail of some work we’ve undertaken so far. The objective is to enable a new wave of systems that exploit emotional and social intelligence for specific applications. It might be keeping professional operators of machinery safe in stressful situations right through to engaging products and services which support true user diversity. If such systems are to work successfully with humans, they must understand complex psychological states. We’re focusing our long-term HMU programme on developing AI-powered systems to detect emotional states in real time – while using cheap and practical sensors.
During the pandemic, the working patterns of my colleagues – as in so many businesses – have evolved to a more flexible model, with prolonged patches of working from home. Our HMU group realised this offered a unique opportunity. We could run long-term experiments from our homes with a persistence hard to match in laboratories, including gathering data around the function of our own brains. This was a prospect we simply couldn’t miss.
A key experiment within the programme is being led by Dom Kelly, a deep learning specialist with a passion for neuroscience. The working days of his team start with the same strange routine: donning an EEG (electro encephalogram) sensor cap, squirting gel into the electrodes, and plugging themselves in. EEG relies on measuring tiny electrical impulses through the skull and so is prone to interference from electronic equipment. Our dedicated screened rooms on the Cambridge Science Park are better than a domestic living room for signal integrity; but a living room is much better for months-long experiments seamlessly scheduled around the remainder of everyone’s day job.
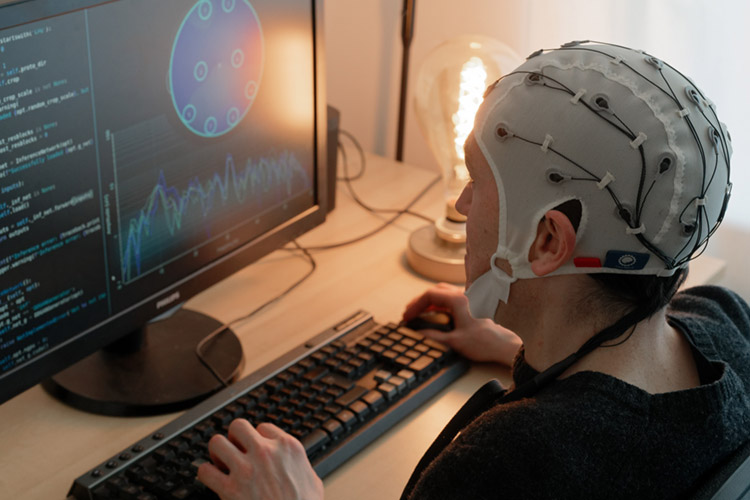
All in a day’s work… deep learning specialist Dom Kelly in his EEG sensor cap
We want to establish how well modern deep learning AI approaches can infer emotional state from basic sensors such as EEG alone. Can they learn to ignore the noise and variability and yet discern whether a participant is watching videos with (say) comforting or disturbing content? Published research in this area is patchy, with little sitting between dubiously bold claims and conventional wisdom on the limitations of EEG and other non-invasive sensors.
AI extracting salient features
We’ve developed an experiment where we randomly play different categories of video clip, while recording EEG traces from an observer. We define each clip we play and its associated EEG traces as a ‘measurement’. Hundreds of measurements are made in a single day, with the experiment continuing to run month after month. This has allowed us to eliminate other variables such as time of day, hunger and so on. With each new measurement, the AI has become better at extracting salient features from EEG traces.
Does this experiment show promise? Yes, but we’re not yet claiming we’ve cracked it. Some runs showed little evidence that the EEG traces encoded the brain’s reaction to the type of video. For example, the first plot below shows how the AI struggles to accurately cluster orange (disturbing content) and blue (anodyne content). There is interesting structure in the data, but it would be hard to define a simple boundary between the two. But on other occasions the results were strikingly clear. The second plot shows how clearly the AI can distinguish which type of content our team member was watching (the green line showing the decision boundary).
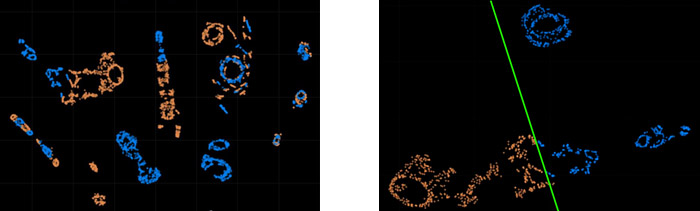
Plot 1: Our AI struggles to separate EEG traces from a participant watching anodyne (blue) or disturbing (orange) video content
Plot 2: This time it clearly distinguishes between a participant watching anodyne (blue) or disturbing (orange) video content
There’s much more to do in this programme, and many hurdles to overcome. Detecting a wider range of emotions, making systems subject-independent… the list goes on. But these results from a basic sensor in real world conditions are exciting. They point to the power of modern AI to make meaningful detections outside of the controlled conditions of a laboratory, enabling a path to ever more scalable and less intrusive deployments. I am both encouraged by progress and optimistic about the coming stages of research.
What will it take for machines to truly understand the emotional human?
So where do we go from here? The next phase of the programme is already taking shape. We’ve targeted a range of objectives, including the synthesis of live images in response to nuances in the EEG signal, as well as closing the loop between the system responding to the user, and the user responding to the system. Please do email me directly if there are any aspects of our initiative that you’d like to discuss or get to grips with further. Let’s keep the conversation going.
—
This article was originally published in Information Age – Unlocking Human-Machine Understanding through real-time emotional state monitoring